Architecture Overview
Architecture Overview
IOMETE is a Data Lakehouse Platform that provides a unified data platform for AI and Analytics. It is built on top of Apache Spark, Iceberg, and Kubernetes. IOMETE is designed to be a scalable, reliable, and high-performance platform for data processing and analytics.
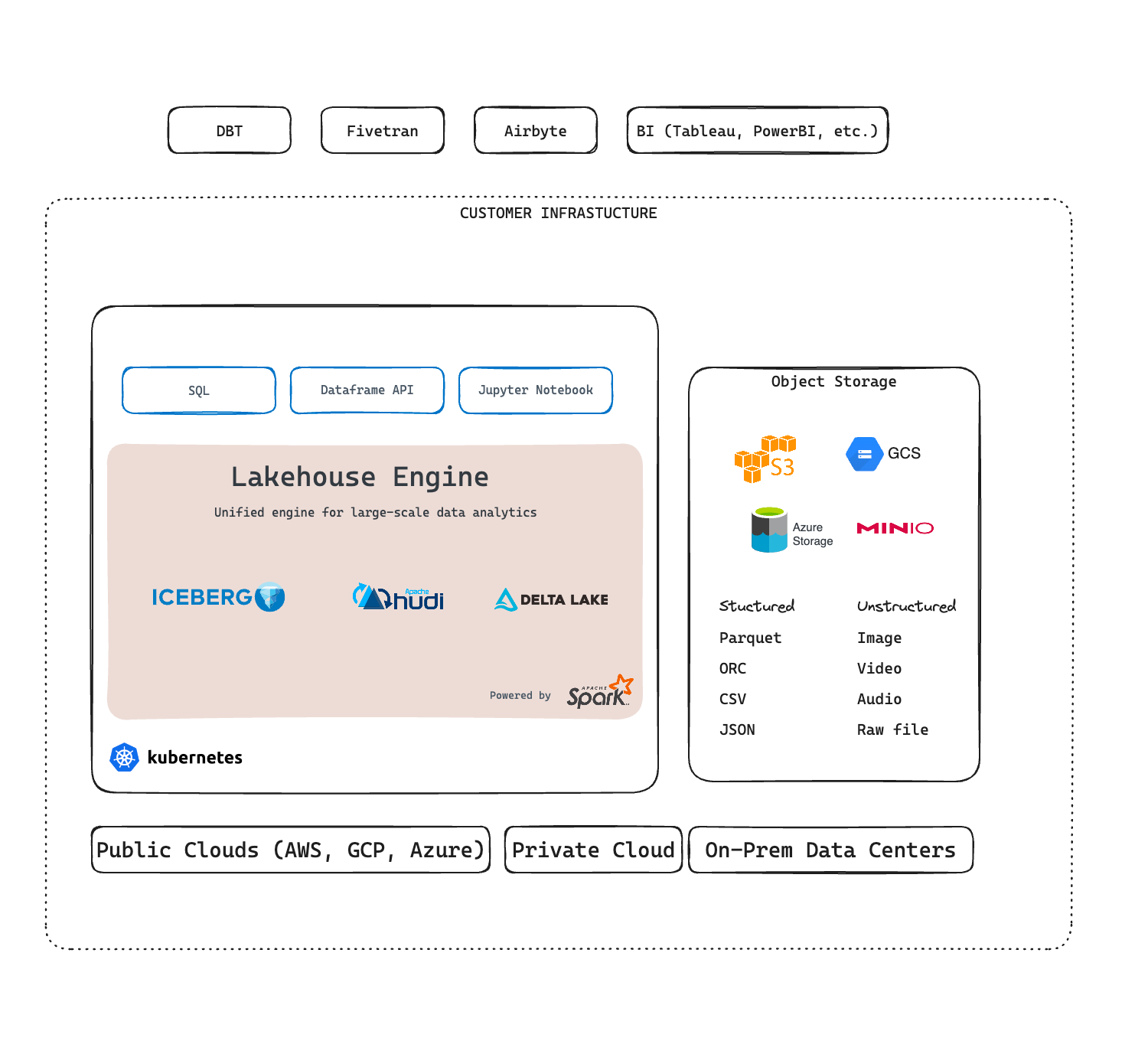
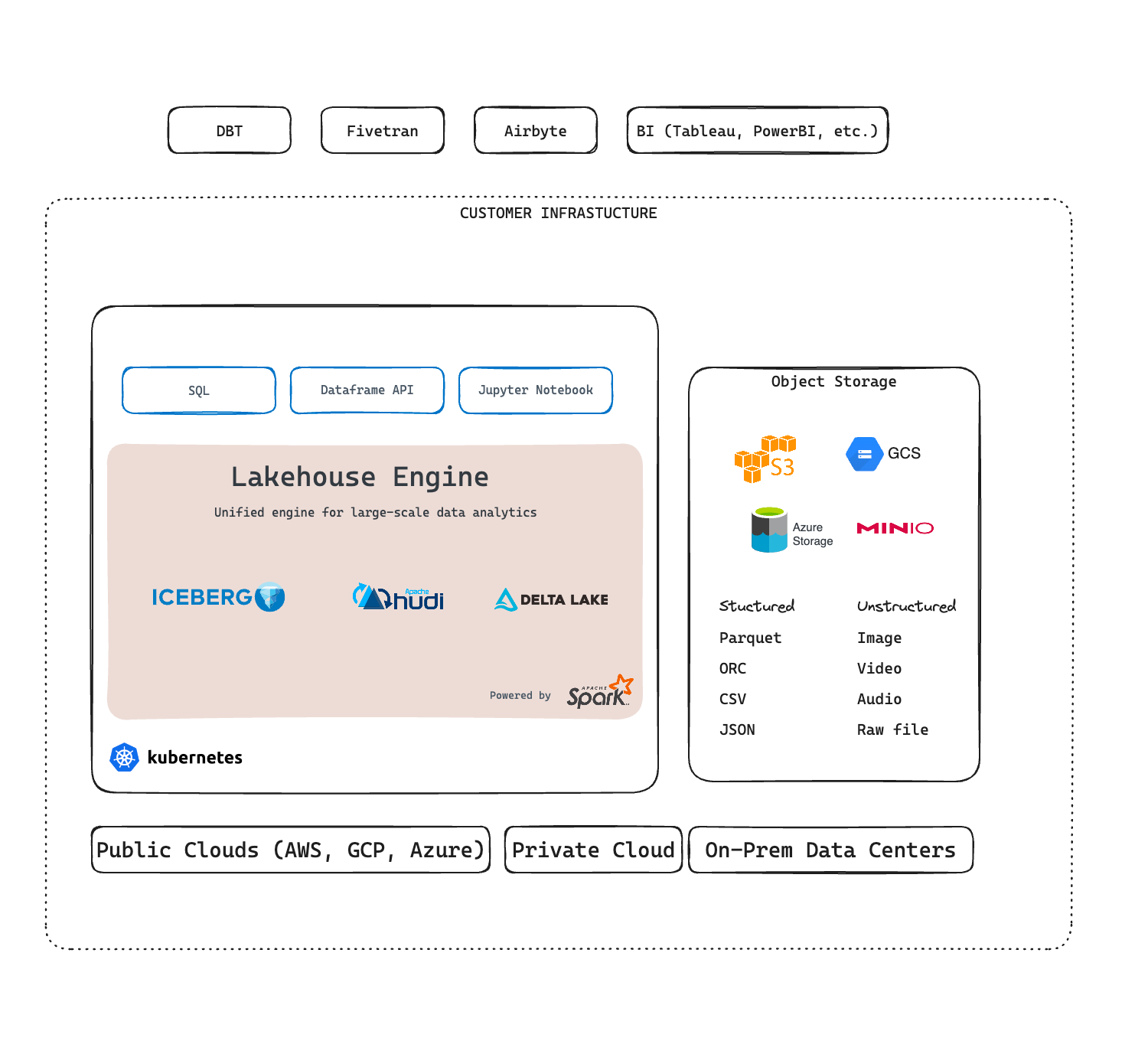
High-Level Architecture
IOMETE runs on top of Kubernetes, which can be deployed on any cloud provider or on-premises data center. IOMETE uses Object Storage (e.g., AWS S3, Google Cloud Storage, Azure Blob Storage, Minio) as the storage layer for data.
Inside Kubenetes, IOMETE is deployed and provides a set of services that are used to manage data, run Spark jobs, and provide a SQL interface to query and process data.
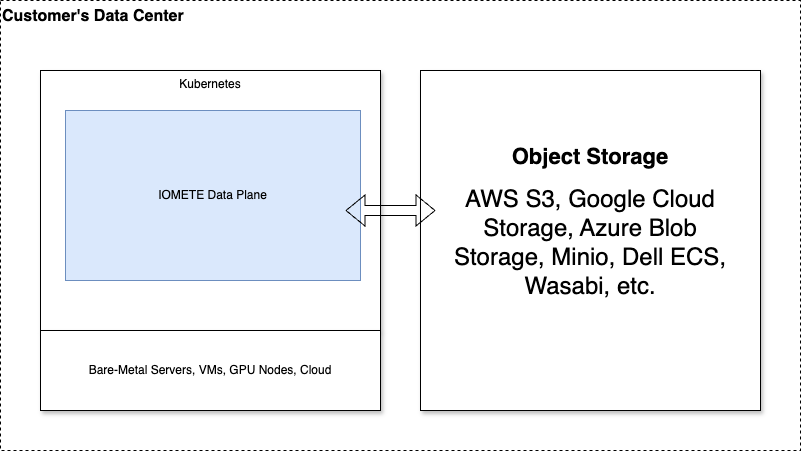
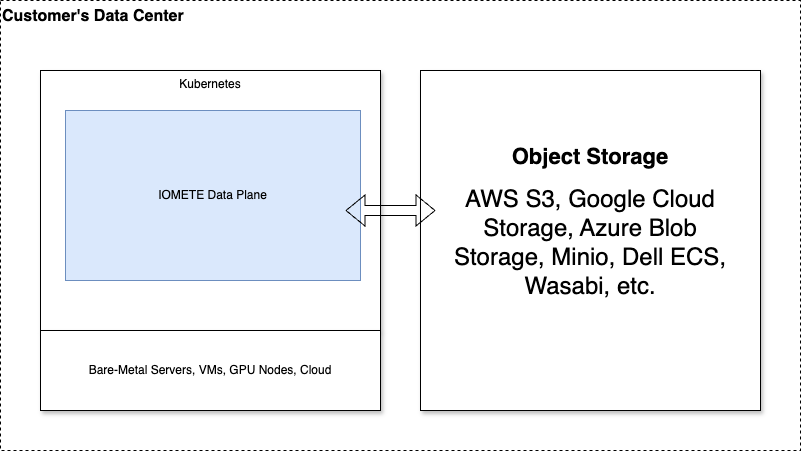
Inside Data Plane - IOMETE Services
If we dive deeper into the IOMETE services, we can see the following components:
- Microservices: IOMETE is built on top of microservices architecture. Each service is responsible for a specific task, such as managing metadata, running Spark jobs, or providing a SQL interface. In the diagram below, bluish boxes represent microservices.
- Workloads: These are the actual workloads based on Spark Engine that serves different purposes.
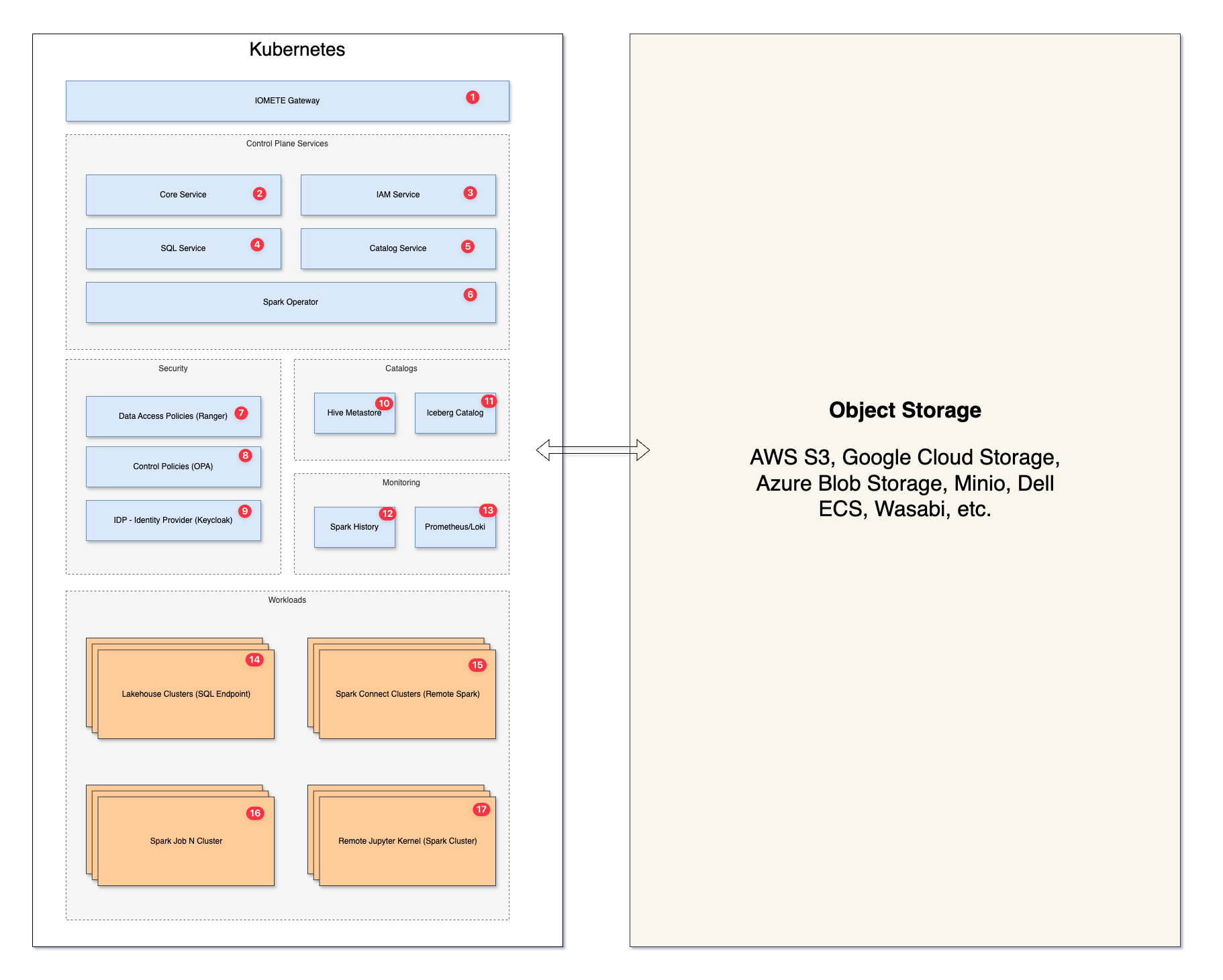
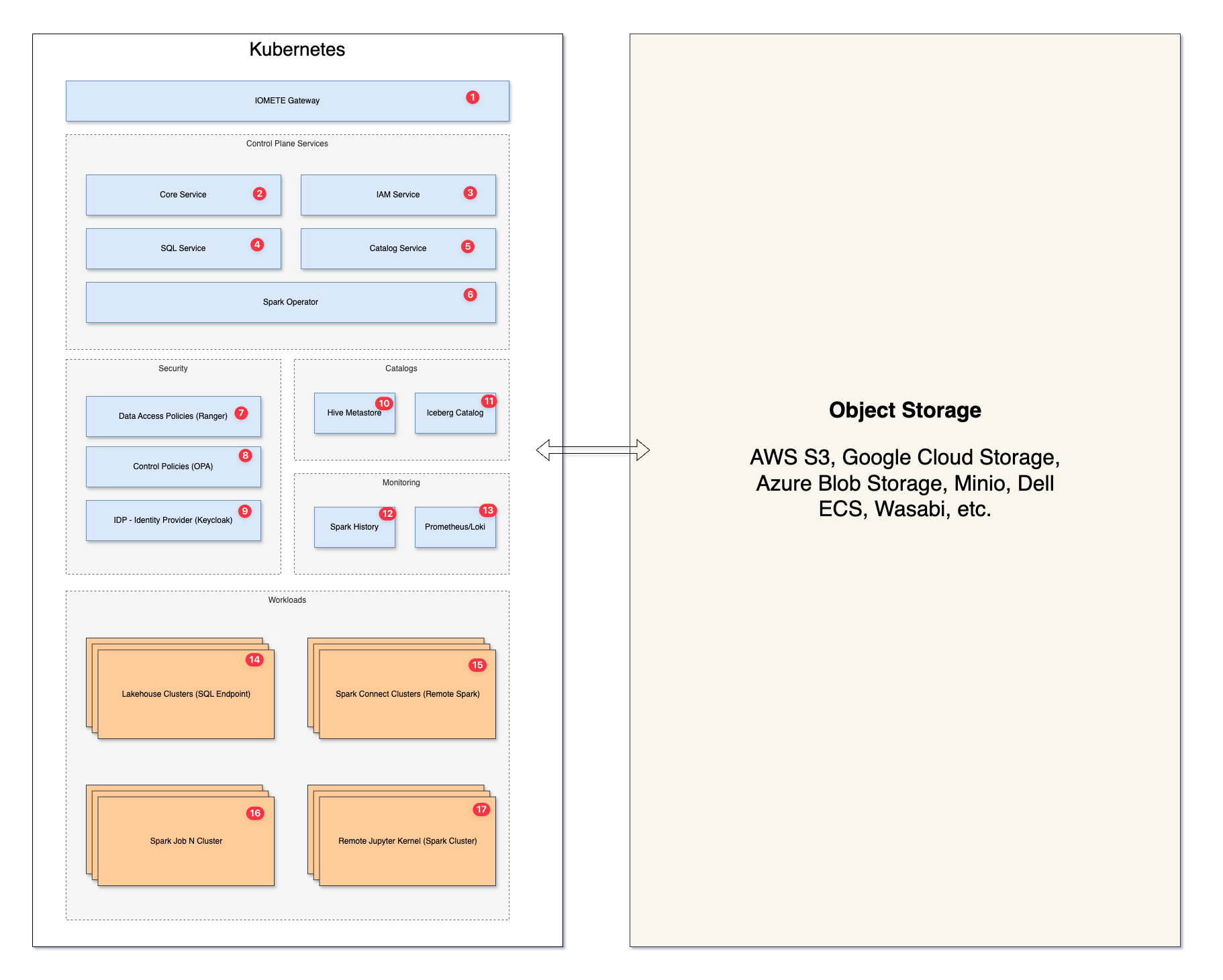
Microservices
1. IOMETE Gateway: IOMETE Gateway is the entry point to the IOMETE platform. It's a reverse proxy that routes requests to the appropriate microservices.
Control Plane Services
- 2. Core Service: Core Service is the main service that manages metadata, scheduling, and orchestration of workloads.
- 3. IAM Service: IAM Service is responsible for managing users, groups, and permissions, as well as managing other IDP integrations, such as LDAP, SAML, etc. See Identity and Access Management.
- 4. SQL Service: SQL Service provides REST API for SQL and also provides a backend for the SQL Editor. See SQL Editor.
- 5. Catalog Service: Catalog Service is a backend service for Data Catalog. Which provides metadata management, data discovery, tagging, and lineage.
- 6. Spark Operator: Spark Operator is a Kubernetes operator that manages all Spark Workloads. It schedules, monitors, and manages the lifecycle of Spark clusters.
Security
- 7. Data Access Policies (Ranger): Data Access Policies is a service that manages data access policies for databases, tables, and columns. It provides a consistent way to manage permissions for groups or users. See Data Security.
- 8. Control Policies (OPA): Control Policies is a service that manages control policies for the platform. It provides a way to manage platform-level policies, such as who can create lakehouses, who can run Spark jobs, etc. See Control Policies.
- 9. IDP - Identity Provider (Keycloak): IDP is a service that manages user storage, authentication, and integration powered by Keycloak.
Catalogs
- 10. Hive Metastore: Hive Metastore is a service that manages metadata for Parquet, CSV, JSON, Hive Tables, etc. It provides a way to store metadata for Hive tables and partitions.
- 11. Iceberg Catalog: Iceberg Catalog is a service that manages metadata for Iceberg Tables.
Monitoring
- 12. Spark History: Spark History is a service that provides Spark job history and monitoring. It's useful for debugging and monitoring completed Spark jobs.
- 13. Prometheus/Loki/Grafana: Prometheus, Loki, and Grafana are services that provide monitoring, logging, and visualization for the platform and workloads.
Workloads
- Lakehouse Clusters: Lakehouse Clusters are the clusters that provide SQL interface that can be connected from any SQL client, BI tools (e.g., Tableau, PowerBI), DBT, or any other tool that supports JDBC/ODBC. See Lakehouses.
- Spark Connect Clusters: Spark Connect Clusters are the clusters that provide remote Spark endpoints. Using the connection, you can connect to Spark from anywhere and execute Spark code (DataFrames, SQL, etc.). See Spark Connect Clusters.
- Spark Jobs: Spark Jobs are the Spark jobs that are submitted to the Spark Engine for execution. See Spark Jobs.
- Jupyter Remote Kernel: Jupyter Remote Kernel is the Jupyter Kernel that can be connected from any Jupyter Notebook to execute code remotely (e.g., ML training, data processing). See Jupyter Remote Kernel.